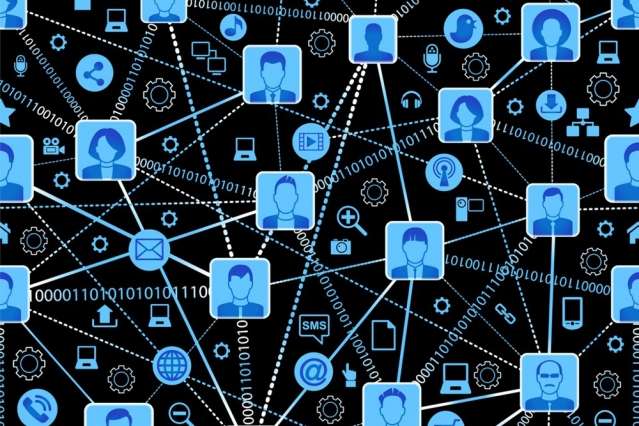
In the course of our day-to-day lives, we produce vast amounts of data. Whether gathered through online communications platforms, tracking devices, or other sources, these data reveal information about our behavior, decisions, and preferences. Researchers can ultimately use the data to improve systems across a variety of domains. In the process, there are great challenges and opportunities in the work of understanding the flow of ideas through groups, determining which incentives are effective, measuring network dynamics, and managing the inherent issues of privacy.
MIT’s Institute for Data, Systems, and Society (IDSS), which aims to advance research at the intersection of engineering and social science, blends multi-disciplinary expertise in systems theory, economics, political science, algorithmic and computational game theory, and network science. Research merging social science with data processing and analysis examines interactions and dynamics over large networks of interconnected individuals—aiming to understand how ideas evolve over networks, quantify the influence of individuals in the networks, and make better predictions.
Understanding and improving the flow of ideas
At the heart of efforts to unravel some of the complexities and implications of social networks is “connection science,” which brings together application and theory. “Connection science is an attempt to actually connect between data, real-world situations, and theory,” says Alex “Sandy” Pentland, the Toshiba Professor of Media Arts and Sciences at MIT and director of the Human Dynamics Laboratory.
“Out of this, comes the notion of the ‘living lab’; rather than having something happen and we record data and only then try to fit theories to it, we’re looking at something that is ongoing, living,” says Pentland. “We can interact with it to understand it better.”
One particular initiative of Pentland and his team looks at how ideas flow in organizations and communities. Pentland and colleagues have been able to identify certain communication patterns that indicate effective collaborations—providing insights into the seemingly ineffable “chemistry” of high-performing groups, companies, and communities. They designed electronic badges and software for mobile phones that reveal characteristics about participants’ interactions with each other. Although the badges and phones don’t measure the content of conversations, they do measure the communications in terms of patterns—such as who is talking to whom and how much people are speaking. After the researchers analyze the data, they can intervene with feedback and incentives—and then determine whether this leads to better ideas and better decisions.
In addition, the researchers are now applying these approaches toward improving education—both in-person and distance learning—determining how to create the most effective interactions. For more information see connection.mit.edu.
Using social data to make predictions and decisions
Devavrat Shah, professor of electrical engineering and computer science, develops statistical inference algorithms guided by behavioral models from social science to extract meaningful information from social data.
“Social data is the data that we all generate,” says Shah, “as a biproduct of things we do.”
Social data can be generated through purchases, reviews, mobile phone traces, censuses, tweets, posts, and interactions on social marketplaces. These data contain a wealth of information that can be utilized for better social living such as social recommendations, informed policy making, efficient business operations, and uplifting societies by, for example, mobilizing untapped labor forces through crowdsourced platforms.
“We have a unique opportunity where as an engineer and social scientist, we can make a huge impact in shaping the future of our society,” Shah says.
In order to realize this opportunity, we need to address the challenge of how to process social data at scale to extract meaningful, accurate information. Shah has been using mathematical models coming from social sciences to develop computationally efficient inference algorithms. For example, to predict trends in Twitter accurately, he and colleagues utilized non-parametric statistical methods along with a classical model for information diffusion in social networks. The resulting algorithm predicted, with 95 percent accuracy, which topics would be trending an average of an hour and a half in advance, and, at times, four or five hours in advance. Similar non-parametric approach, when married with different behavioral model, leads to efficient prediction algorithm for price of Bitcoin. This resulted in a profitable trading strategy. Using a statistical model—suggested by practitioners Dawid and Skene in 1979—Shah and colleagues developed algorithms based on graphical models to design economically efficient low-cost crowdsourcing system. The resulting algorithm is utilized in peer-grading platforms for online education and various citizen science projects.
“Understanding human choice is foundational,” Shah says. “It is at the core of our ability to predict the consumer demand, the foundational concept of macroeconomics. In a democratic society, it determines the way we govern. And in modern times, it is what determines how we receive online recommendations and advertisements.”
Shah and colleagues have developed computationally efficient statistical methods for learning the “discrete choice model” from sparse data. This collection of works have resulted into novel ranking (or election) algorithm based on comparison data, recommendation systems and efficient decision-making for business operations. This work is an excellent example, where behavioral models from social science inspired new development in statistical inference. Shah co-founded Celect, which has been commercializing research of Shah and colleagues.
Applications to policy in the developing world
These approaches are also implemented to understand how well policies and programs in developing countries are performing—and how they can be improved. Esther Duflo, the Abdul Latif Jameel Professor of Poverty Alleviation and Development in the Department of Economics, and colleagues in the Abdul Latif Jameel Poverty Action Lab (J-PAL) use different types of randomized-control trials to gather data to help determine to what extent certain social policies are achieving their objectives.
“In our work, we are interested in causal effects of a policy or intervention, or sometimes someone’s characteristics, for example their education, on an outcome or a series of outcomes,” says Duflo. “I’m never trying to look at ‘What is the entire model of someone’s behavior?’ I’m always interested in looking at ‘What is the effect of a particular cause that is, in principle, manipulatable or changeable?'”
Duflo and colleagues evaluate a wide variety of different programs and policies aimed at reducing poverty, including microfinance initiatives—trying to determine whether there are data to indicate they are beneficial, and also determine whether there are hindrances in the programs’ efficacy. Participation in a microfinance program may be highly variable, and might depend on different dynamics and interactions within networks, or communities, of people. Duflo and her team developed a model of “word-of-mouth diffusion” and then applied it to data on social networks and participation in a newly available microfinance loan program in 43 Indian villages. The model allowed researchers to distinguish information passing among neighbors from direct influence of neighbors’ participation decisions, as well as information passing by participants versus nonparticipants. The model estimates suggest that participants are seven times as likely to pass information compared to informed nonparticipants, but information passed by nonparticipants still accounts for roughly one-third of eventual participation.
In addition to understanding how well a socio-economic program or policy is working—or not working—and why, data can also be used to understand whether a program can be successfully replicated in other areas of the world. J-PAL is able to evaluate the same program, applied in different areas, at the same time. For example, a comprehensive program designed for Bangladesh was also evaluated in six more countries. The effects were mostly positive, in varying degrees, across the entire the population in the study.
Understanding and predicting sociopolitical change
Complex questions related to political change, cultural dynamics, and societal transformation require an innovative new set of theory, modeling, field experiments, and algorithms. Understanding and predicting sociopolitical change requires a new set of tools and a multidisciplinary analytical framework.
The Department of Defense Multi-Investigator University Research Initiative (MURI) project brings together a team of researchers to address this challenge. This major collaboration involves a number IDSS principal investigators, including: Munther Dahleh, Daron Acemoglu, Fotini Christia, Munther Dahleh, Ali Jadbabaie, and Asuman Ozdaglar. They have developed a framework to study collective action and collective decisions, including how local interactions among individuals and groups with different information, levels of prominence, and preferences results in the spread of information and actions. By developing theories of cascade and contagion in conjunction with field surveys and experiments, IDSS PIs are investigating social and political changes in societies (such as Arab Spring events), using theories and a wide range of datasets ranging from online social networks such as Twitter, Facebook, and LinkedIn, to data from Afghanistan, Iraq, and Yemen.
For example, as part of this project, researchers analyzed three years’ worth of cellphone call metadata from Yemen—January 2010 to October 2012—to determine the effect of events such as drone strikes and protests on call patterns. The data also provide valuable insights into Yemeni culture and day-to-day life. For example, the research has provided clues to effect of drone strikes on movement patterns and social ties and have opened up a window to the study of the effect of such shocks on how people communicate and how news of such events spreads. In other parts of this project, the PIs have investigated issues related to collective decision making and collective action, specifically, questions about how individuals make decisions by combining individual observations and opinions of others and how social cascades occur.
“This project is really an example of what IDSS is all about: It involves data, systems, and societal elements,” says Ali Jadbabaie, associate director of IDSS. “It brings together political scientists, economists, systems theorists, data scientists, and computer scientists to address important societal questions.”
[Source:- Phys org ]